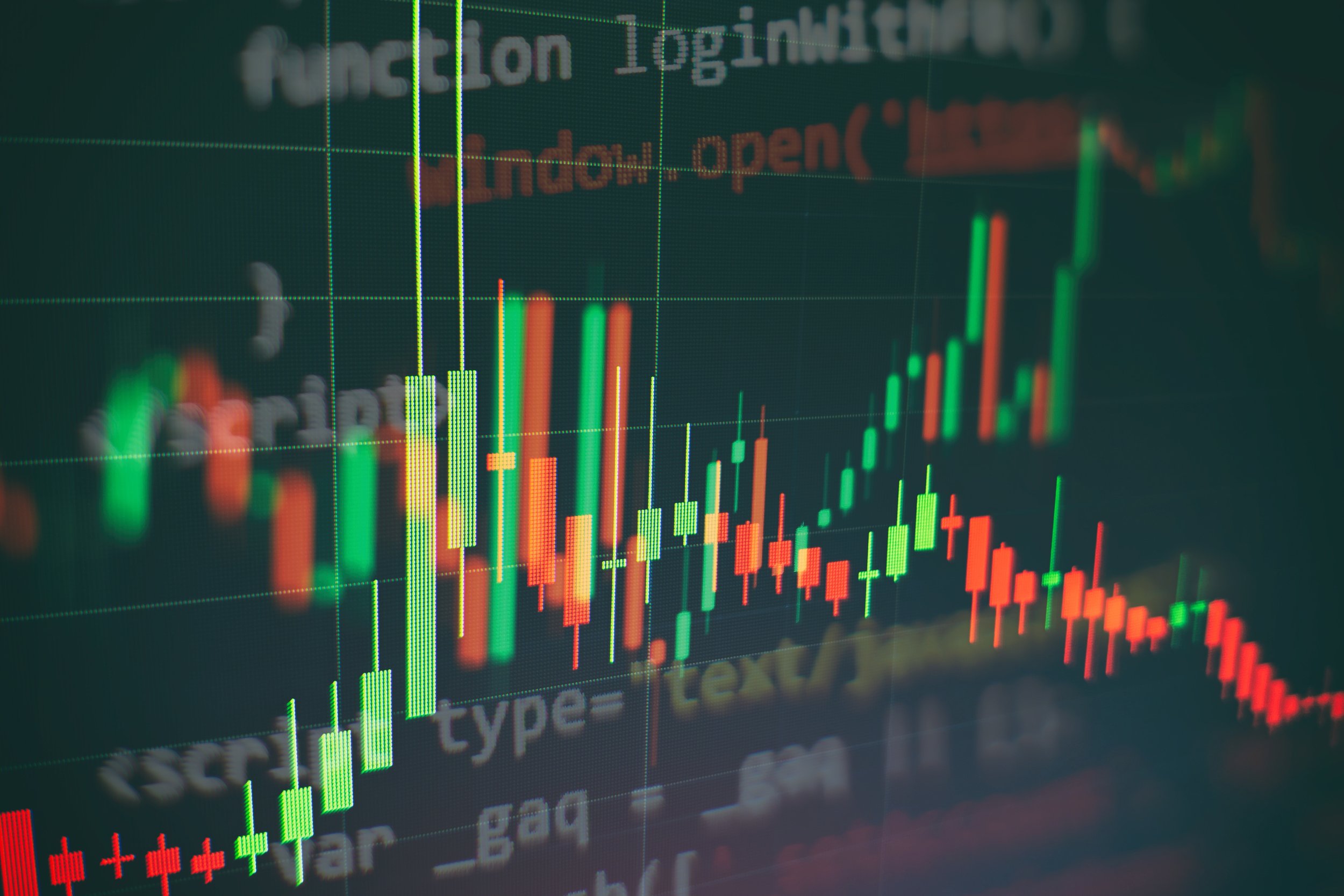
Statistical Analysis
Statistical analysis encompasses a set of tools and procedures used to find patterns and differences in the data as well as relationships between variables. Statistical analysis involves collecting and analyzing large amounts of data to identify common patterns and trends, and converting data into insightful information.
We have experience designing dashboards and tracking Key Performance Indicators (KPIs). We deliver descriptive analytics to various audiences in the form of intuitive, user-friendly charts, graphs, and tables. Besides producing inferential analysis, predictive analysis, and prescriptive analysis, our expertise also lies in correlation analysis, regression analysis, and analysis of variance (ANOVA).
Descriptive Analytics
Descriptive analytics summarize and highlight patterns in current and historical data. We use descriptive analytics to identify trends and to describe relationships between variables. Realizing the importance of monitoring and reporting performance, we use descriptive analytics to inform on progress toward goals and key performance indicators (KPIs). We are able to track metrics, glean insights from survey and focus group data, and identify trends in consumer preference and behavior.
Correlation Analysis
Correlation is used to test relationships between variables. We use correlation analysis to identify the relationships, patterns, significant connections, and trends between variables of interest. We have employed correlation analysis in projects involving data mining, socio-economic research, and market research.
Regression Analysis
Regression analysis is a technique used to model and analyze the relationships between variables and how they contribute to producing an outcome. We use regression analysis to study the causal relationships between the independent (predictor) and dependent (predicted) variables. Depending on the type of dependent and independent variables, dimensionality in the data, and other factors, we employ different types of regressions to assess the strength of the relationship between variables, and to predict and forecast. The value of using regression analysis is that it helps an organization determine which factors matter most, how those factors interact with each other, and how they may affect or impact an outcome.
Analysis of Variance (ANOVA)
In some cases, sample data can be divided or partitioned into various groups. Some situations require that we examine whether a total sample can be considered homogenous or not. As such, we use analysis of variance (ANOVA) to determine whether differences between groups of data (within a total sample) are statistically significant. We analyze the levels of variance within groups through samples taken from each of them. We have used ANOVA in projects involving how a policy has affected different groups within a sample. We have also conducted ANOVA to understand different customer groups’ experience with the same product.